Zico Kolter: OpenAI's Newest Board Member on The Biggest Questions and Concerns in AI Safety | E1197
04 Sep 2024 (8 months ago)
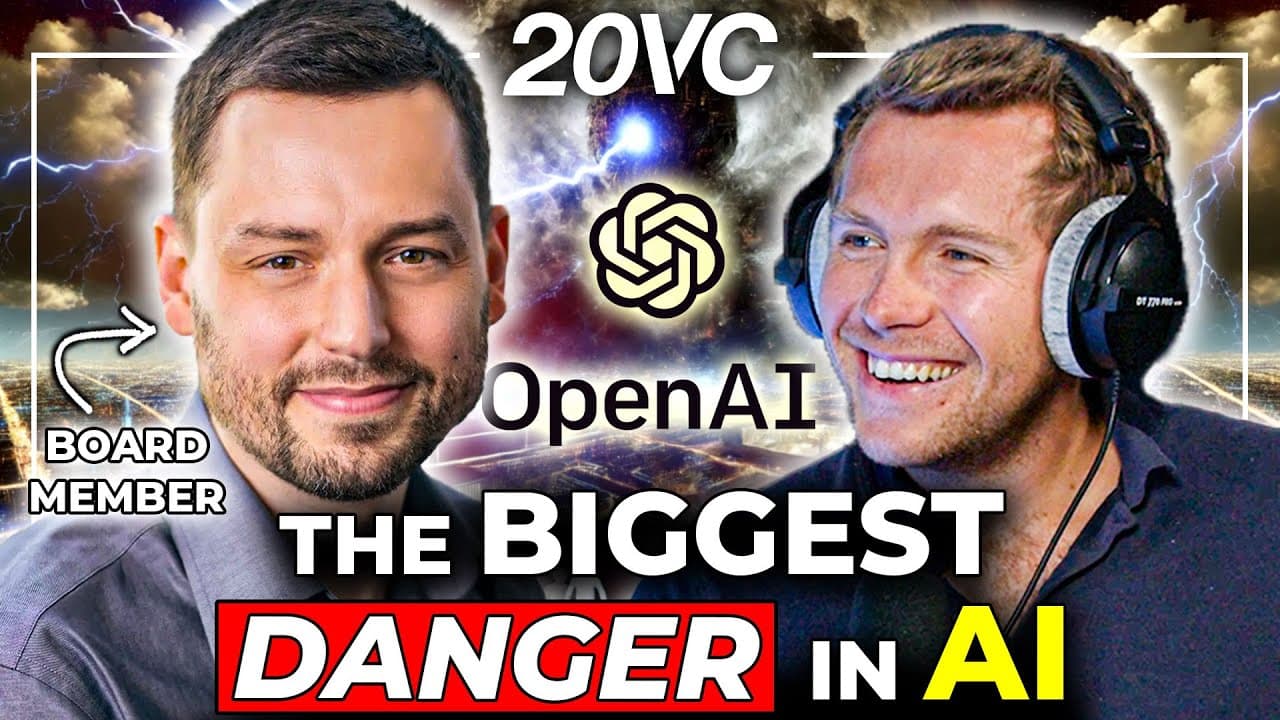
Intro (0s)
- The pervasive spread of misinformation makes people question the validity of everything they encounter, even without AI's influence, although AI accelerates this trend. (0s)
- Humans are biologically predisposed to trust information from close associates, a tendency rooted in our evolutionary history. (16s)
- Zico Kolter holds multiple positions, including Professor and Head of the Machine Learning Department at Carnegie Mellon University, a role he has held for 12 years. (54s)
Understanding the Basics Behind Modern AI Technology (1m29s)
- Large language models (LLMs) are trained on vast amounts of internet data to predict the next word in a sequence. (1m52s)
- The process involves building mathematical equations that learn to predict words based on the preceding words in a given text. (2m2s)
- Despite the seemingly simple mechanism of predicting words, LLMs exhibit intelligence by generating coherent and contextually relevant responses. (3m39s)
Data Availability & Synthetic Data (4m17s)
- There is a large amount of data that is not publicly available and is not being used to train models. (5m51s)
- Current models are limited by the amount of data they can process, not by the amount of data that is available. (7m0s)
- Video data is much larger than text data, making it more difficult to process. (7m16s)
Why AI Performance Doesn't Plateau Despite Data Limits (9m8s)
- Increasing the size of AI models continues to lead to performance improvements, even with fixed datasets. (9m47s)
- Current AI algorithms do not extract the maximum possible information from the data provided, suggesting significant room for improvement in data processing and utilization. (10m51s)
- The perception of plateauing gains in AI models is attributed to users' limited imagination in exploring the full potential of these models, rather than limitations inherent in the models themselves. (16m1s)
How Will AI Models Evolve Amid Rapid Commoditization (16m14s)
- The rapid commoditization of AI models, which were once expensive and limited to a few players, is changing the AI landscape. (16m14s)
- While many companies are currently focused on training their own models, this may not be economically viable in the future, potentially leading to consolidation in the industry. (17m30s)
- Although some argue that the industry has reached a point of diminishing returns with increased compute power, scaling laws suggest that compute remains a significant factor in improving AI models, even if there might be more efficient approaches. (18m11s)
Are Corporations Pursuing AGI or Profitable AI Products? (19m9s)
- AGI is defined as a system that can function as well as a close collaborator on a year-long project. (19m39s)
- Large enterprises are hesitant to use cloud-based AI for training due to concerns about data mobility, transferability, and access rights. (23m12s)
- There are misconceptions about how AI models are trained, with some believing that any data used in a query is also used for training the model, which is not true. (25m18s)
The Danger of Misinformation & Lack of Trust in Objective Reality (27m55s)
- The proliferation of misinformation and deepfakes is a significant concern, potentially leading to widespread distrust in any information encountered. (28m53s)
- Humans have not always had access to objective records of information, and relying on trusted sources within close circles is a return to a more natural state of information dissemination. (29m50s)
- While AI accelerates the spread of misinformation, it did not invent the concept, and existing social, economic, and governmental structures can potentially be adapted to regulate its impact. (36m43s)
The Concerns and Hierarchy of Safety in AI (37m14s)
- The most pressing concern in AI safety is the inability of current AI models to reliably follow specifications, making them susceptible to manipulation and misuse. (37m29s)
- This vulnerability, akin to a buffer overflow, poses a significant risk as AI models are integrated into larger systems and interact with untrusted data, potentially granting control to malicious actors. (39m43s)
- The potential harms of AI models, including cyberattacks, bio-risks, and the creation of harmful artifacts, are amplified by this vulnerability, making it crucial to address the issue of AI models reliably following specifications. (42m58s)
The Considerations of Releasing Open-Source Models (44m45s)
- Open-source models have been instrumental in advancing AI research and are a critical part of the AI ecosystem. (46m47s)
- There is a concern that releasing highly capable models open-source could have negative consequences, particularly if they are used for malicious purposes, such as finding vulnerabilities in software. [2978]
- There is a need to prioritize AI safety now, focusing on practical concerns like preventing misuse and ensuring the safety of critical infrastructure, rather than solely focusing on far-fetched scenarios like rogue AI. (57m4s)
Quick-Fire Round (59m10s)
- The speaker, previously focused on model architectures, now believes they are less important, suggesting any architecture can be successful with enough effort. (59m42s)
- Initially believing in the need for highly curated data, the speaker now acknowledges the power of using vast amounts of readily available internet data for training AI models. (1h0m27s)
- The speaker expresses concern about the overemphasis on AI architectures, like the Transformer, and hopes to shift the focus towards data and capabilities of AI models. (1h3m7s)