Liquid AI's Ramin Hasani on liquid neural networks, AI advancement, the race to AGI & more! | E1928
10 Apr 2024 (1 year ago)
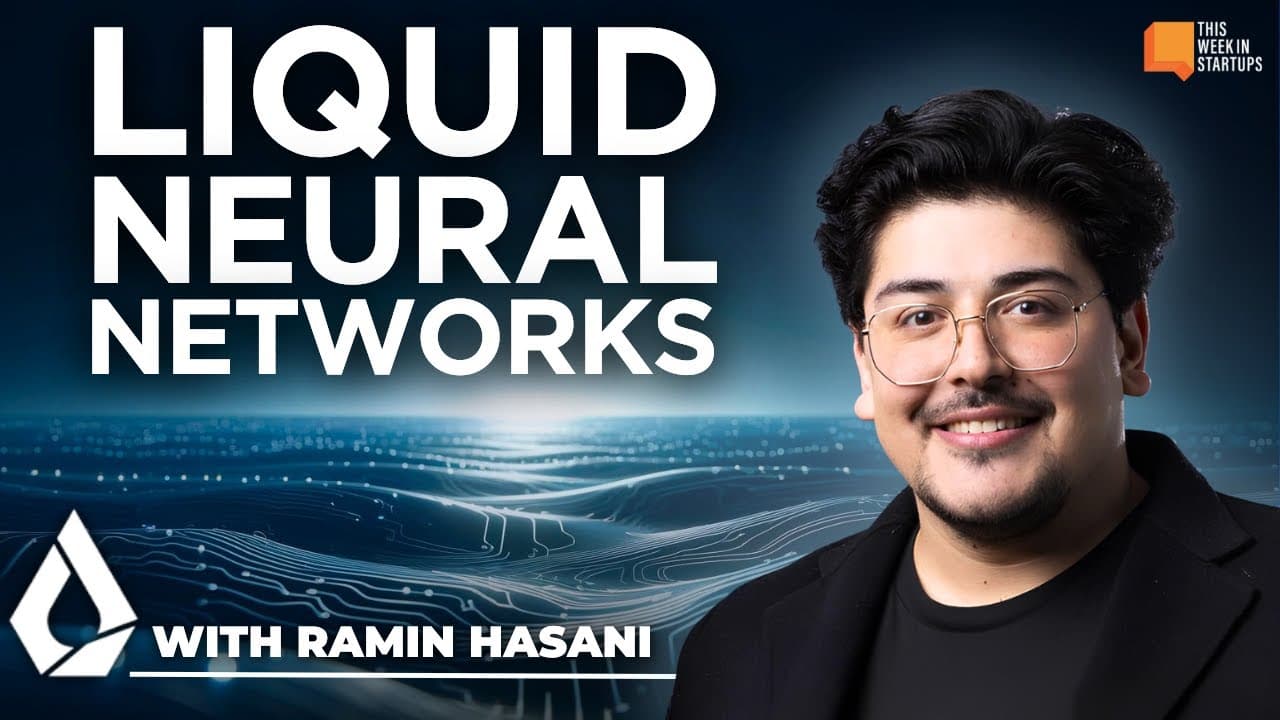
Liquid AI CEO and co-founder Ramin Hasani joins Jason (0s)
- Ramin Hasani, CEO and co-founder of Liquid AI, joins Jason.
- Artificial general intelligence (AGI) has the potential to solve major global challenges such as the energy crisis, economic issues, and political structures.
- AGI could lead to the creation of the most valuable company on Earth.
- The race to develop AGI is underway, but it is unlikely that all companies will reach AGI at the same time.
- Some companies are expected to achieve AGI before others.
Liquid AI's mission and concept of liquid neural networks (1m9s)
- Liquid AI, developed by Ramin Hasani, aims to design artificial intelligence systems based on biological and physical principles, inspired by the nervous system of the worm C. elegans.
- Unlike traditional AI systems, liquid neural networks are differentiable, allowing for continuous error propagation and learning.
- Liquid neural networks use continuous signals, unlike the human brain's spiking neurons, which are not yet fully understood.
- Liquid neural networks exhibit adaptability, adjusting to new inputs even after training, resulting in more dynamic, real-time, and robust responses compared to fixed AI systems with trained weights.
- Ramin Hasani is the CEO and co-founder of Liquid AI.
- Liquid AI is a company that develops AI software for natural language processing and understanding.
- Liquid neural networks are a new type of neural network that is more efficient and powerful than traditional neural networks.
- Liquid neural networks are inspired by the human brain and can learn and adapt more quickly than traditional neural networks.
- Liquid neural networks are being used in a variety of applications, including natural language processing, image recognition, and robotics.
- AI is advancing at an exponential rate.
- AI is expected to have a major impact on our lives in the coming years.
- AI is being used in a variety of fields, including healthcare, finance, and transportation.
- The race to develop artificial general intelligence (AGI) is heating up.
- AGI is a type of AI that is capable of matching or exceeding human intelligence.
- AGI is expected to have a profound impact on our world.
- The future of AI is bright.
- AI is expected to revolutionize many aspects of our lives.
- AI has the potential to make the world a better place.
Demo of Liquid AI: traditional vs. liquid neural networks in autonomous driving (8m34s)
- Liquid neural networks, inspired by the brain of the worm Caenorhabditis elegans, have fewer parameters and are more robust to noise compared to traditional neural networks.
- In an autonomous driving task, a liquid neural network with only 19 neurons and 1,000 parameters outperformed a traditional neural network with 500,000 parameters.
- Liquid neural networks have the potential to be much smaller and more efficient than traditional neural networks, making them suitable for applications with limited resources.
- Liquid AI's Ramin Hasani discusses liquid neural networks and their potential to revolutionize AI by capturing fundamental principles of neural computation.
- By studying simpler organisms like worms, scientists aim to scale up these principles and build advanced AI systems that surpass existing capabilities.
Practical applications of Liquid AI (16m7s)
- Liquid AI systems have been used to successfully drive cars autonomously and fly drones.
- The US Air Force has demonstrated the use of Liquid AI to fly F-16 jets.
- Liquid AI systems excel at modeling time series data, including video, audio, text, user behavior, financial time series, and medical time series.
- Liquid AI systems are general-purpose computers that can perform sequential decision-making processes.
- Liquid AI has the potential to change the spectrum of how AI is done today.
- Time series is a series of similar data points over time.
- Examples of time series include stock prices, steering wheel alignment, and vehicle speed.
- Liquid neural networks are particularly good at studying time series data.
- Audio signals and video data can be considered time series.
- Language is a sequential kind of data, but the time element is different.
- Ramin Hasani, CEO of Liquid AI, discusses liquid neural networks, AI advancements, and the race to achieve artificial general intelligence (AGI).
- Liquid neural networks are a new type of neural network that is inspired by the human brain.
- They are more flexible and efficient than traditional neural networks, and they can be used to solve a wider variety of problems.
- Liquid neural networks are still in their early stages of development, but they have the potential to revolutionize the field of artificial intelligence.
- AI is advancing at an exponential rate.
- In the past few years, we have seen major breakthroughs in natural language processing, computer vision, and robotics.
- AI is now being used in a wide variety of applications, from self-driving cars to medical diagnosis.
The Race to AGI (20m7s)
- The race to achieve AGI is one of the most important challenges facing the scientific community.
- AGI is the hypothetical ability of a machine to think and reason like a human being.
- Achieving AGI would have a profound impact on our world, and it could lead to new technologies that we can't even imagine.
Commercializing worm-inspired AI systems, building a team, and solving problems across various sectors (21m37s)
- Liquid AI, founded by Ramin Hasani, Maas Lechner, Alexander Amini, and Danielus, aims to develop practical AI systems to solve real-world problems.
- The company has secured $42 million in seed funding at a $300 million valuation.
- Liquid AI is building a team of experts from prestigious institutions to work on liquid neural networks, an alternative to traditional transformer-based AI systems.
- Their goal is to create an efficient and accessible AI infrastructure applicable across various industries, including finance, biotechnology, and autonomous systems.
- Liquid AI offers an enterprise-facing product with a developer package, allowing businesses to utilize its high performance.
- The models can be developed on the edge, and even run on a small and inexpensive computing unit like a Raspberry Pi, showcasing the technology's efficiency and accessibility.
Efficiency of liquid neural networks in compact devices like the Raspberry Pi and the transformative potential of AI modeled after worms. (27m30s)
- Liquid AI, led by Ramin Hasani, introduces liquid neural networks, an energy-efficient and scalable alternative to Transformer models.
- Liquid AI has partnered with system integrators like Capgemini, Itochu CTC, EY, and Accenture to commercialize their platform across various industries.
- Liquid neural networks claim to be 10 to 1,000 times more efficient for inference and 10 to 20 times more efficient for training compared to current models, potentially reducing AI development costs.
- While the AI community focuses on scaling up Transformer models, Liquid AI takes a different approach by changing the fundamental architecture and building new frameworks.
- Liquid neural networks can scale more efficiently than Transformers, achieving similar performance with fewer parameters.
- Liquid AI plans to train very large liquid neural network models after securing additional funding.
- Liquid AI's models challenge the dominance of Transformer models and provide an alternative path for scaling AI systems.
- Liquid AI currently utilizes Nvidia GPUs for training but requires significantly fewer GPUs compared to other large language models.
- Ramin Hasani is the CEO and co-founder of Liquid AI.
- Liquid AI is a company that develops liquid neural networks, a new type of neural network that is more efficient and powerful than traditional neural networks.
- Liquid neural networks are inspired by the human brain.
- They are made up of interconnected nodes that can process information in parallel.
- This allows them to learn faster and perform better on tasks that require a lot of computation, such as image recognition and natural language processing.
- Liquid AI believes that liquid neural networks are the key to advancing AI.
- They are working on developing new liquid neural network architectures and algorithms.
- They are also working on making liquid neural networks more accessible to researchers and developers.
- Liquid AI is one of many companies that are racing to develop artificial general intelligence (AGI).
- AGI is a type of AI that is capable of performing any intellectual task that a human being can.
- Liquid AI believes that liquid neural networks are the key to achieving AGI.
- Liquid AI believes that AI has the potential to revolutionize the world.
- They are committed to developing AI that is safe, beneficial, and accessible to everyone.
- They believe that AI can help us solve some of the world's most pressing problems, such as climate change and poverty.
Data ownership in AI and incentivizing data providers (35m24s)
- Liquid AI's Ramin Hasani proposes a licensing arrangement where data providers receive compensation for their data usage in AI systems, similar to content creators on social media platforms.
- Liquid AI's developer package is available to clients for solving AI problems, such as predicting surgical phases from video data.
- Artificial General Intelligence (AGI) is defined as a system capable of performing any intellectual task a human can.
- AGI could exist in virtual environments like the internet.
- The debate surrounding AGI focuses on its potential capabilities and limitations.
Role of AI in real-world applications (41m18s)
- AI is making progress in virtual applications like answering legal questions, marketing plans, writing, chess, and verticalized games.
- For AI to translate into the real world, it needs embodiment, such as robotic arms, computer vision, and fine motor skills.
- There are already robotic applications in the real world, such as strawberry picking.
- However, AI still lacks the fine motor skills needed for complex tasks like playing basketball or soccer.
- AGI is defined as the ability to beat a human at any task.
- The next 2-5 years are expected to bring significant leaps in AI performance as models grow in size.
- With 100 trillion parameters, AI could reach human-level capacity.
- This level of advancement may be achieved within the next 2-5 years, resulting in an AI that feels smarter than any human and can beat any human in any test.
Societal impact of AI, job displacement, and the optimistic view on AI's potential (43m57s)
- Liquid AI's Ramin Hasani believes that AI will revolutionize industries, enhance human capabilities, and positively impact society.
- AI can assist humans in various tasks, making them more productive and efficient.
- AI has the potential to solve complex scientific and mathematical problems, leading to advancements in physics, mathematics, and humanitarian sciences.
- AI can address existential problems like clean energy, farming, and healthcare, creating a world of abundance.
- While AI may displace some jobs, it can also create new opportunities and allow humans to focus on more fulfilling work.
- Explainability in AI is crucial, as it allows people to understand how AI systems make decisions.
Explanation of physical models vs statistical models in AI and the challenge of understanding black box AI systems (50m25s)
- Liquid AI's Ramin Hasani emphasizes the importance of explainability in AI systems, contrasting physical models' full explainability with statistical models' lack thereof.
- Current AI systems are often black boxes due to their unexplainable nature.
- Mechanistic interpretability and causal models provide some understanding of system behavior, but liquid neuron networks offer a better grasp of input-output behavior.
- Liquid AI aims to develop understandable and efficiently deployable AI systems using explainable math.
- The AI industry's focus on speed and monetization over understanding and explaining AI decision-making creates a misalignment of incentives that hinders the progress of explainable and aligned AI systems.
Speculations about AGI's market cap, who might achieve AGI first, and the potential of using AI systems to build various applications (57m1s)
- The market cap of AGI could be equivalent to the value of the entire world.
- Companies that develop AGI could become the most valuable on Earth.
- OpenAI and Anthropic are likely to be the first to achieve AGI.
- Both companies are focused on scaling AI systems into more powerful entities.
- The release of large language models like Claude and OpenAI's API will present challenges and require careful rollout and testing.
- The recent chaos at OpenAI might be due to the company feeling that its next version of its AI system is approaching AGI.
Comparison between open-source and closed-source models in AI and the trend of open-source moves in the AI industry (1h0m12s)
- Closed-source models receive more resources and concentrated effort compared to open-source models.
- The gap between closed-source and open-source capabilities is growing.
- Some companies, like Facebook, Apple, and Mr. Al, are moving towards open-source models, but closed-source models still dominate.
- Open-source models often have delayed releases or are released with limited capabilities compared to their closed-source counterparts.
- Liquid AI is hiring and has a team of around 25 highly talented individuals.
- The team includes Olympiad medalists, inventors of important AI technologies, and philosophers.
- Liquid AI values bringing in key players to build and scale meaningful AI solutions.