Stanford ECON295/CS323 I 2024 I The AI Awakening, Erik Brynjolfsson
14 Aug 2024 (9 months ago)
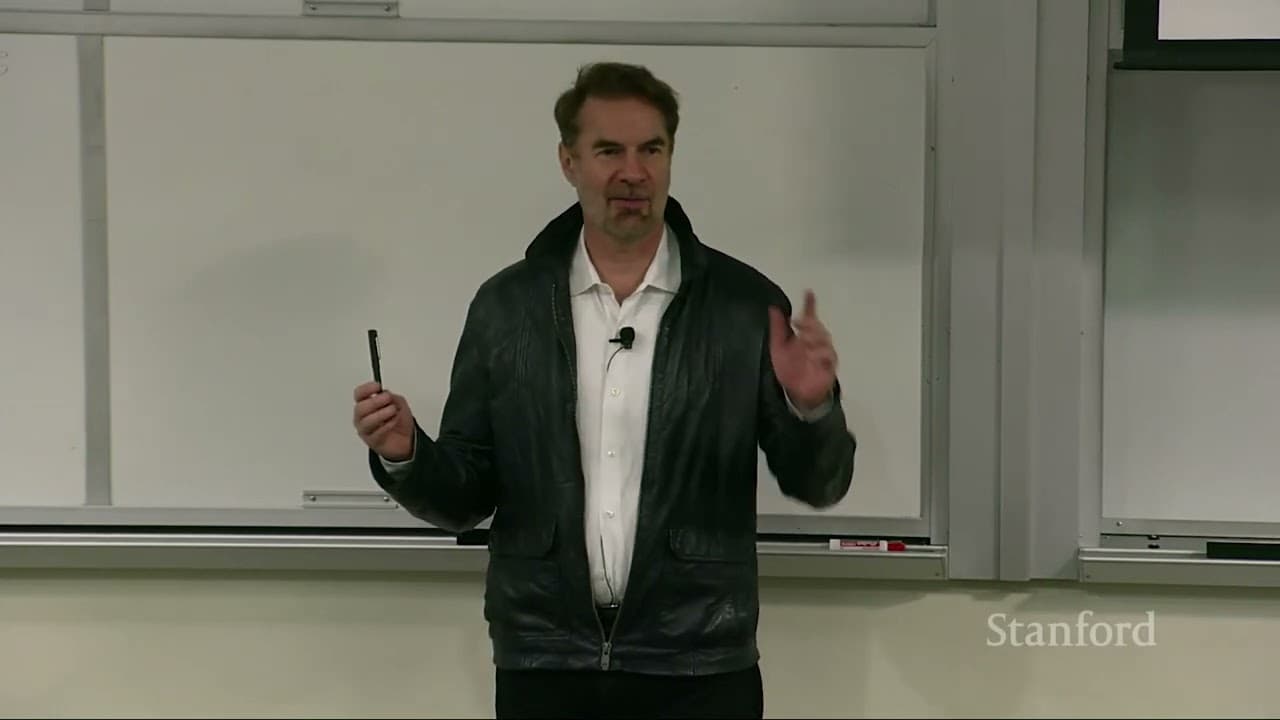
AI Progress and Impact
- The speaker, Eric Brynjolfsson, begins the class by asking students if they believe AI progress is accelerating and having a greater impact on the economy and society.
- Most students agree, citing the recent buzz around large language models like ChatGPT.
Factors Driving AI Progress
- One student, Tyler, suggests that the increased access to computing power and infrastructure is a major factor driving AI progress.
- Tyler argues that the availability of more powerful computers allows for the training of larger and more complex AI models, leading to a self-fulfilling cycle of investment and improvement.
- The speaker identifies three key factors driving the AI revolution: increased computing power, a vast increase in digital data, and improved algorithms.
- The speaker highlights the importance of the transformer, a recent invention, in managing and utilizing data more effectively.
AI's Impact on Society
- Another student notes that while the technology itself may be improving, there is also a perception that AI is having a broader impact on society.
- This student suggests that AI's potential to affect all industries, unlike previous technologies that were often limited to specific sectors, contributes to this perception.
- The impact of AI on consumers is becoming more apparent, particularly with the rise of accessible AI interfaces like chatbots.
- While there is a perception that AI is revolutionizing the workforce, the actual impact on the economy is currently limited, with generative AI software revenues estimated at only $3 billion in 2023.
The "Bitter Lesson" and Data-Driven Progress
- The "Bitter Lesson" by Richard Sutton argues that progress in AI is primarily driven by increased data and compute power, rather than algorithmic advancements.
- The rapid advancement of AI technology, particularly in the field of large language models, is attributed to the availability of more computing power and data.
- The use of large language models has led to significant improvements in language understanding, demonstrating the effectiveness of learning from vast amounts of data.
- Chris Manning, a prominent figure in natural language processing, acknowledges the progress made in AI's ability to understand language.
The Potential of Synthetic Data
- The speaker raises concerns about the potential for a data shortage, as current AI models have been trained on nearly all available data, including scraped internet content and books.
- The speaker acknowledges the possibility of using synthetic data to address this data shortage, but questions its effectiveness.
- AlphaGo was trained on human play games, while AlphaZero was trained on zero human data.
- AlphaZero was able to generate its own games and learn from them, demonstrating the potential of synthetic data for training AI.
- The speaker suggests that there are certain problems that are well-suited for training with synthetic data, such as games with well-defined rules, while others may be more difficult.
AI's Potential and Concerns
- The speaker expresses concern about the potential for AI to malfunction in unexpected ways, highlighting the need for further research and understanding.
- The speaker acknowledges the hype surrounding AI and the prevalence of unfounded claims, but emphasizes that there is a genuine technological revolution underway.
- AI researchers have been surprised by the recent advancements in AI capabilities, indicating a significant inflection point in the field.
- The speaker believes that the rapid improvement in AI technology will lead to significant economic changes, but worries about the lack of corresponding changes in business institutions and culture.
The Importance of Understanding GPTs
- The speaker highlights a growing gap between economic understanding and the rapid advancements in technology, particularly in the realm of artificial intelligence (AI).
- He emphasizes the importance of bridging this gap to address the challenges and opportunities presented by AI in the coming decade.
- The speaker proposes that understanding the impact of general-purpose technologies (GPTs) is crucial for navigating these changes.
- He defines GPTs as technologies that are pervasive, improvable, and capable of spawning complementary innovations.
- The speaker cites the steam engine as the first GPT, followed by electricity and computers.
- He argues that AI qualifies as a GPT, possessing all the characteristics of previous GPTs.
- The speaker acknowledges that the internet, while impactful, may not be considered a GPT due to its lack of a similar "J-curve" effect on living standards as seen with the steam engine and electricity.
- He emphasizes the importance of understanding how GPTs, including AI, will reshape economics, business processes, and institutions.
AI's Potential for Solving Global Problems
- Artificial intelligence (AI) is a general-purpose technology that can be applied to various fields, including healthcare, poverty, and consumer goods.
- Demis Hassabis, the founder of DeepMind, believes that solving intelligence can lead to solutions for various global problems.
The Evolution of AI
- The field of AI has evolved from symbolic methods to rule-based systems and now to deep learning techniques.
- The early stages of AI research focused on symbolic methods due to limited computational power.
- In the 1980s, expert systems were developed using hand-coded rules.
- Machine learning emerged as a new approach to AI, replacing the traditional method of explicitly programming instructions.
- Instead of providing specific instructions, machine learning utilizes data on inputs and outputs to identify statistical relationships and make predictions.
- Examples of machine learning applications include anti-money laundering, handwriting recognition, and credit scoring.
The Rise of Generative AI
- Generative AI, also known as Foundation Models or Large Language Models (LLMs), represents a new era in AI that builds upon machine learning.
- LLMs utilize unsupervised or self-supervised learning, which requires less human annotation compared to traditional machine learning.
- LLMs are trained through a process of predicting the next word or token in a sequence, based on the preceding words.
- This process is similar to supervised learning but does not require human labeling of data, as the data itself provides the necessary information.
- Large language models, such as the transformer model, can be trained on massive amounts of data, such as trillions of words.
- These models can predict the next word in a sequence, which allows them to generate text.
- The models can be adjusted to generate text that is more or less plausible.
- To predict the next word, the models need to have some understanding of the world, including grammar, concepts, and relationships.
- Similar techniques can be used to generate images by filling in missing parts of images.
- This self-supervised approach allows for rapid learning from data.
Generative AI's Capabilities and Challenges
- Generative AI models are becoming increasingly capable, surpassing expectations in some areas, such as passing the bar exam.
- Generative AI models require a large amount of data to train, raising questions about how to compensate data contributors.
- Generative AI models are unexpectedly good at a variety of tasks, including writing, translating, and coding.
- GPT-4 performed better than 90% of humans on the Uniform Bar Exam, demonstrating significant progress in AI capabilities.
The Potential for General AI
- The text discusses the progress of large language models (LLMs) and their potential for achieving general artificial intelligence (AI).
- The author mentions a chart showing a correlation between increased compute power, data set size, and parameters with improved LLM performance.
- The author notes that while this trend suggests significant progress, the cost of achieving such advancements could become unsustainable due to the exponential increase in required resources.
- The author references the Metaculus website, which hosts predictions on various topics, including AI milestones.
- The author highlights that predictions for the arrival of general AI have shifted significantly closer in recent years, with the estimated date moving from 2075 to 2031.
- The author suggests that this accelerated timeline is due to unexpected progress in LLMs and the potential for embodied AI, which is considered a crucial aspect of general AI.
AI's Economic Impact and Distribution of Benefits
- The speaker expresses skepticism about the immediate development of humanoid robots, but acknowledges the potential of large language models (LLMs) for robotics.
- Yan Lun, an economist, believes that while AGI may not be imminent, powerful AI systems can still have a significant transformative effect.
- Yan Lun initially considered LLMs a dead end, but later acknowledged their potential for economic value, stating that they could generate trillions of dollars in impact.
- The speaker emphasizes that while AI can boost productivity and increase economic output, it does not guarantee equitable distribution of benefits.
- The speaker cites the example of declining wages for those with high school education or less, despite overall productivity growth, as evidence of potential economic disparities.
AI as a Complement to Human Capabilities
- The speaker criticizes the Turing test as a measure of intelligence, arguing that it focuses on replicating human behavior rather than evaluating true intelligence.
- The speaker suggests that AI research should focus on developing technologies that complement human capabilities rather than simply replacing them.
- The speaker argues that throughout history, most technologies have acted as complements to human labor, increasing its value rather than replacing it.
- The speaker cites the example of a person with a bulldozer or computer being able to create more value than someone without these tools, demonstrating how technology amplifies human capabilities.
The Misguided Focus on Automation
- The speaker mentions Alan Turing and Neil Nelson, who shared a vision of creating human-level intelligence by automating tasks that humans perform.
- The speaker suggests that this vision, while energizing technologists and business executives, may be misguided as it focuses on replicating existing tasks rather than creating new value.
- The speaker uses the example of Deus, a mythological Greek inventor, and Karel Čapek, who coined the term "robot," to illustrate the historical fascination with creating human-like machines.
- The speaker argues that simply automating existing tasks, even if done perfectly, would not significantly improve living standards.
- The speaker emphasizes that most improvements in living standards have come from new products, services, and inventions, not just from replacing human labor.
The Potential for Infinite Productivity and its Implications
- The speaker highlights the concept of productivity as output divided by input, and points out that if labor hours go to zero, productivity would theoretically become infinite.
- The speaker suggests that while infinite productivity might seem desirable, it could lead to a situation where labor income goes to zero, potentially impacting political power.
- The speaker discusses the potential for infinite productivity through AI, but cautions that it could lead to wealth and power concentration if not managed carefully.
- The speaker emphasizes the importance of finding alternative ways to distribute wealth and maintain political power in a world where human labor is less essential.
AI's Impact on the Workforce
- The speaker presents a case study of a call center that implemented an AI system to provide suggestions to human operators.
- The AI system, trained on call center transcripts, identified patterns and provided suggestions to operators, leading to a 14% increase in accuracy and efficiency.
- The speaker notes that the AI system had a greater impact on less skilled workers, resulting in a 35% productivity improvement for them, while more skilled workers saw minimal improvement.
- The speaker acknowledges the concern that AI could displace workers, but argues that AI is better at handling common problems with abundant data, while humans are better at handling unique or rare situations.
- The speaker suggests that the line between what AI can handle and what humans are better at is constantly shifting, and that there is a natural division of labor between humans and AI.
- The speaker discusses a study on call centers that used large language models (LLMs) to augment human operators. The study found that the use of LLMs resulted in increased productivity, improved customer satisfaction, and higher employee satisfaction.
- The speaker mentions that the study's findings suggest that augmentation with AI may be a viable path forward for many tasks.
- The speaker cites a paper by Daniel Rock and colleagues at OpenAI that analyzed 18,000 tasks in the US economy and found that at least 10% of the tasks performed by 80% of the workforce could be affected by AI.
The Evolution of Human-AI Collaboration
- The speaker discusses the six levels of self-driving cars, noting that the same levels could apply to other tasks in the economy.
- The speaker uses the example of chess to illustrate how the relationship between humans and AI has evolved. Initially, humans and AI could work together to outperform the best AI systems. However, AI has progressed to the point where humans have little to offer in terms of skill or strategy.
- The speaker shares his personal experience with Tesla's self-driving technology, expressing skepticism about its reliability and noting that he remains vigilant while driving.
Unanswered Questions and Future Directions
- The speaker concludes by posing a question about the types of problems that AI is currently unable to solve.
Course Structure and Requirements
- The course has required readings, typically two to four per week.
- Students can submit questions via Slido, an automated system that allows for voting on questions.
- The course includes weekly assignments and a team project.
- Teams must be formed by April 12th and should be diverse in terms of program affiliation.
- The course has two progress reports and two sessions at the end of the class, one for policy/research proposals and one for business plans.
- The course is graded on a 20% basis for each category: readings, assignments, team project, and participation.
- The course features a lineup of speakers, including Erik Brynjolfsson.
- Students can work alone or in teams on the team project.
- Teams should be diverse in terms of program affiliation, with members from computer science, economics, business, engineering, and other programs.
Discussion Sections and Guest Speakers
- The speaker discusses the benefits of having a diverse class, acknowledging that it can be more challenging to teach but ultimately provides a valuable experience.
- The speaker encourages students to take advantage of the opportunity to work with people from different programs at Stanford.
- The speaker explains that optional discussion sections are structured around specific topics, with Teaching Assistants (TAs) leading some sessions and other volunteers contributing.
- The speaker mentions that the first discussion session will focus on introductions, while the second will be for team formation.
- Future discussion sessions will cover topics such as writing a business plan and government policy, with guest speakers invited to share their expertise.