Why Vertical AI Agents Could Be 10X Bigger Than SaaS
22 Nov 2024 (7 months ago)
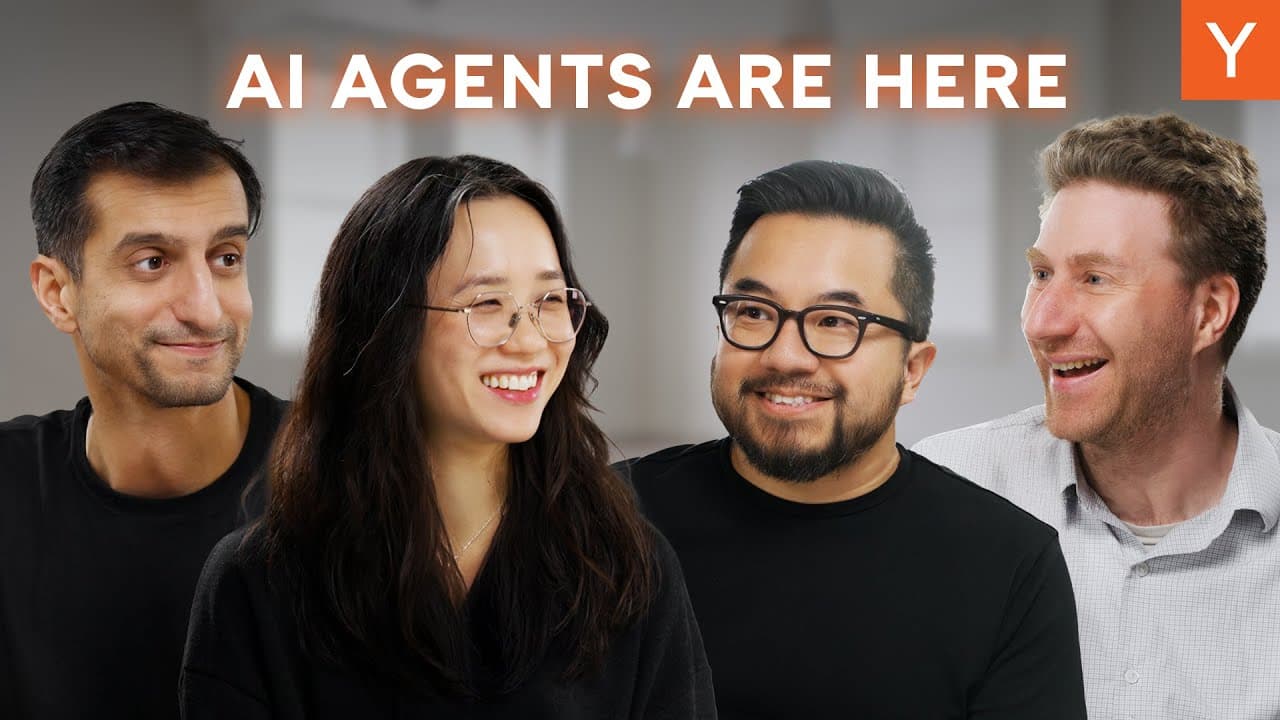
Coming Up (0s)
- The progression of AI has been rapid, with significant improvements every 3 months, leading to the development of full-on vertical AI agents that can replace entire teams and functions in enterprises (7s).
- The current state of AI is still surprising, with the potential for vertical AI agents to have a major impact (11s).
- Foundation models are becoming increasingly competitive, with multiple players emerging to challenge the dominance of Open AI (17s).
- The increasing competition in the AI market is beneficial, as it provides consumers with choices and gives founders opportunities to succeed (30s).
- The hosts of the episode, Gary, Jared Harge, and Diana, have collectively funded hundreds of billions of dollars worth of startups, often investing in companies when they were just starting out with one or two people (52s).
Jared is fired up about vertical AI agents (1m1s)
- Vertical AI agents are expected to be a significant market, with potential for $300 billion plus companies, but many people, especially young startup founders, are not fully appreciating their potential (1m9s).
- The concept of vertical AI agents is not new, but the world has not yet caught on to their potential, with some people already talking about and funding them (1m22s).
- The growth of vertical AI agents can be compared to the growth of SaaS, which was also underestimated by many people, especially young startup founders, who tend to see the startup industry through the lens of the products they use as consumers (1m49s).
- SaaS has been a significant part of Silicon Valley's funding for the last 20 years, with over 40% of venture capital dollars going to SaaS companies, resulting in over 300 SaaS unicorns (2m16s).
- The real catalyst for the SaaS boom was the introduction of XML HTTP request in 2004, which enabled the creation of rich internet applications in web browsers (2m45s).
- This technology shift allowed software to move from being installed on desktops to being used through websites and on phones, creating the SaaS boom (3m15s).
- Paul Graham was one of the first people to realize the potential of XML HTTP request, using it to create the first SaaS app, ViaWeb, an online store similar to Shopify, in 1995 (3m29s).
- The early SaaS apps were not successful due to the lack of XML HTTP request, but the technology improved over time, leading to the growth of SaaS (3m50s).
- The current AI technology, specifically LLM, is seen as a new computing paradigm that makes it possible to do something fundamentally different, similar to the impact of XML HTTP request on SaaS (4m8s).
- The growth of cloud and mobile technologies in 2005 led to a big open question about what to do with the new technology and where the value would accrue, leading to the creation of many billion-dollar companies (4m20s).
- The different paths taken by these companies can be bucketed into categories, leading to a realization about the potential of vertical AI agents (4m38s).
- There are three categories of startup ideas: obviously good ideas that could be mass consumer products, mass consumer ideas that were not obvious, and B2B SaaS companies. (4m43s)
- The first category includes products like Google Docs, photos, email, calendar, and chat, where zero startups won and 100% of the value went to incumbents like Google, Facebook, and Amazon. (4m54s)
- The second category includes companies like Uber, Instacart, DoorDash, Coinbase, and Airbnb, which were not obvious and came out of left field, allowing startups to win in those spaces. (5m30s)
- The third category, B2B SaaS companies, has created the most billion-dollar companies, with over 300 companies in this space, including Salesforce, which is considered the first true SaaS company. (6m0s)
- Mark Benioff, the founder of Salesforce, initially faced perception issues when trying to build sophisticated enterprise applications over the cloud or via SaaS, as people believed that real software had to be bought in a box. (6m37s)
- The early web app and SaaS story is reminiscent of today's situation with AI tools, where people doubt the ability to build sophisticated enterprise applications using these tools due to their limitations. (7m6s)
The parallels between early SaaS and LLM’s (7m25s)
- The parallels between early SaaS and LLMs (Large Language Models) can be seen in the categories of mass consumer applications, which are huge opportunities but likely to be dominated by incumbents, such as general-purpose AI voice assistants (7m27s).
- Incumbents like Apple, Google, and others will compete to be the dominant player in these categories, but may not necessarily win due to the innovator's dilemma, where they prioritize protecting their existing business over pursuing new opportunities (7m49s).
- The innovator's dilemma is exemplified by Google's dominance in search, but the rise of new players like Perplexity, which may challenge Google's position (8m2s).
- Incumbents may be hesitant to pursue new opportunities due to regulatory risks, as seen in the cases of Uber and Airbnb, which were initially considered risky and potentially threatening to existing businesses (8m14s).
- The founders of Uber, such as Travis, took significant personal risks, including the possibility of going to prison, to build their company, which is unlikely to be replicated by highly paid employees of incumbent companies (8m56s).
- The reluctance of incumbents to pursue new opportunities can create space for new players to emerge and disrupt existing markets, as seen in the cases of Uber and Airbnb, which were not cloned by incumbents even after they became successful (8m41s).
Why didn’t the big companies go into B2B SaaS? (9m9s)
- The reason big companies didn't go into B2B SaaS is that it's too hard for them to do many things, as each B2B SaaS company requires people who are extremely deep in one domain and care about obscure issues (9m20s).
- B2B SaaS companies need to be specialized in a specific domain, which is difficult for large companies to achieve, as seen in the example of Gusto, a payroll company that Google didn't build a competitor for due to the complexity of payroll regulations (9m42s).
- The unbundling of software is another reason why big companies didn't dominate B2B SaaS, as it's easier for them to focus on a few huge categories rather than trying to own everything (10m2s).
- The shift to SaaS and the internet has enabled the emergence of vertical B2B SaaS products, which can provide a better user experience than traditional enterprise software (10m23s).
- Traditional enterprise software, such as Oracle and SAP, often has a poor user experience due to trying to cover too much ground and being a "jack of all trades, master of none" (11m8s).
- Vertical B2B SaaS companies can provide a 10x better experience than traditional enterprise software due to their focus on a specific domain (11m24s).
- There are only a few price points in software, which map directly to consumer, SMB, or enterprise sales, and enterprise software is often terrible because it's not the user buying it, but rather a high-up executive (11m37s).
How employee counts might change (12m25s)
- The number of employees in a company often scales with revenue, but this trend might change with the use of large language models (LLMs), as they can automate tasks and reduce the need for hiring (12m25s).
- In the past, companies reaching $100-200 million in revenue would typically have 500-2,000 employees, but with LLMs, this might not be the case, and companies might be able to grow with fewer employees (12m47s).
- The advice given to companies is shifting, with a greater emphasis on hiring software engineers who can understand and work with LLMs to automate tasks and reduce costs (13m28s).
- This shift could result in a significant change in the way startups grow their businesses, with a focus on building LLM systems that bring down costs and reduce the need for hiring (14m8s).
- There is a possibility that a future unicorn company could be run with only 10 employees, with the use of LLMs allowing for greater efficiency and automation (14m26s).
- This trend is not new, as some companies have already seen the benefits of using engineers to automate tasks, such as Mike, the founder of a company that built a smart frying pan, who used his engineering skills to scale up marketing efforts (15m6s).
- The use of engineers to automate tasks can lead to greater leverage and efficiency, and LLMs can take this even further, allowing companies to achieve more with fewer employees (16m17s).
The argument for more vertical AI unicorns (16m25s)
- There is potential for 300 vertical AI agent unicorns, equivalent to the number of SaaS unicorns, with each SaaS unicorn having a corresponding vertical AI unicorn in a new universe (16m25s).
- The vertical AI equivalent of a SaaS company is a product that combines software and people, offering a more comprehensive solution (16m55s).
- Enterprises are currently unsure about what AI agents they need, and one approach is to let them deploy and customize AI agents for their specific needs (17m1s).
- Experienced founders, such as Brett Taylor, the CTO of Facebook, are working on platforms that enable Enterprises to deploy and customize AI agents (17m8s).
- Companies like Vector Shift are building platforms to make it easy for Enterprises to build their own internal LLM-powered agents using no-code or SDKs (17m39s).
- Enterprises often don't know exactly what they want to use AI agents for, and this uncertainty may lead to the development of general-purpose AI platforms before vertical AI agents (17m50s).
- The history of SaaS shows that consumer applications worked first, and this may not be the case for AI agents, as Enterprises have already been trained on the value of point solutions and vertical solutions (18m30s).
- The user experience of AI agents may not be significantly different from existing software, and Enterprises may be willing to take a bet on startups promising good vertical AI agent solutions (19m11s).
- There is evidence that companies are getting faster traction in Enterprises for vertical AI agents than ever before, indicating that the market is still in its early stages (19m34s).
- The development of software often starts with vertical solutions and becomes more developed over time, suggesting that vertical AI agents may follow a similar pattern (19m45s).
- Companies often start by creating specific point solutions, but as they grow, they need to expand horizontally, increasing spending on sales and marketing to continue growing, and eventually, they need to offer more comprehensive solutions that work together to maintain growth (20m2s).
- The bull case for vertical AI agents is that they could be even bigger than SaaS, as SaaS still requires an operations team or people to operate the software and complete workflows, whereas vertical AI agents could replace not only the SaaS software but also a significant portion of the payroll (20m32s).
- Vertical AI agents could disrupt SaaS companies by being 10 times larger, as they would need fewer humans to perform tasks such as data entry or approvals, making smaller companies more efficient and reducing the need for a large payroll (21m14s).
- The potential for vertical AI agents to be larger than SaaS companies lies in their ability to automate tasks and workflows, reducing the need for human intervention and increasing efficiency (21m16s).
- The growth of vertical AI agents could lead to a scenario where smaller companies can be more efficient and successful with fewer employees, disrupting the traditional model of large companies with many employees (21m20s).
Current examples of companies/uses (21m31s)
- Vertical AI agent companies are gaining traction, with examples including Outset, which uses large language models to improve surveys and qualtrics, and MCH, an AI agent for QA testing, which is seeing great traction and has the potential to replace QA teams entirely (21m32s).
- These types of businesses often need to navigate the challenge of selling to key decision-makers in organizations, who may be hesitant to adopt AI solutions that could replace their teams, and must therefore sell to higher-level executives who are less likely to be threatened by the technology (22m15s).
- Traditional Enterprise and SMB software sales often focus on a particular person or team, but vertical AI agents can be sold top-down, requiring CEO sign-off, and can be more effective in replacing certain teams or functions (22m20s).
- Companies like Rainforest QA, which offered QA as a service, faced challenges in scaling and growing due to the tension between selling software that made QA teams more efficient and replacing those teams entirely, but AI-powered solutions like MCH can now replace QA teams entirely, simplifying the sales process (23m11s).
- The pitch for MCH is not to make QA teams faster, but to eliminate the need for a QA team altogether, allowing the company to focus on selling to engineering teams and companies without large QA teams (23m56s).
- Vertical AI agent companies like MCH have the potential to be 10 times bigger than SaaS companies, as they can scale with companies and replace entire teams or functions, rather than just making them more efficient (24m25s).
- Similar challenges and opportunities exist in other areas, such as recruiting, where AI-powered solutions can replace certain functions or teams, but require careful navigation of the sales process to avoid threatening key stakeholders (24m31s).
- The use of AI in various industries is becoming more prevalent, with companies like A Priori and Cap.ai building AI-powered tools that can handle tasks such as technical screening and developer support, reducing the need for human recruiters and support teams (25m13s).
- A Priori is a company that uses AI to handle the full technical screen and initial recruiter screen, and is gaining traction in the market (25m20s).
- Cap.ai has built a chatbot that can respond to technical details and ingest developer documentation, YouTube videos, and chat history, allowing companies to have smaller developer support teams (25m49s).
- The customer support AI agent market is crowded, but most companies are only doing simple zero-shot LLM prompting that cannot replace a real customer support team (26m46s).
- To replace a customer support team, companies need more complicated software that can handle complex workflows, and there are only a few companies attempting to do this (27m18s).
- The market for customer support AI agents is still open, with only a few companies having less than 1% market penetration (27m30s).
- Hyper-specialization or hyper-verticalization is likely to occur in the customer support AI agent market, with companies focusing on specific industries or use cases rather than trying to build a general-purpose solution (27m35s).
- Companies like Gig.ml are building AI-powered customer support agents that can handle specific tasks, such as handling 30,000 tickets per day for companies like Zepto, and replacing teams of thousands of people (27m58s).
- The concept of vertical AI agents could be 10 times bigger than SaaS, as customers require heavily tailored solutions that are hard to build for everyone, leading to a $300 billion SaaS market instead of a $1 trillion meta SaaS market (28m36s).
- Different verticals, such as customer support, require unique kinds of support and training sets, making it challenging to create a one-size-fits-all solution (28m55s).
- The problem of every company growing to a point where it becomes inefficient to be larger is known as Coase's theory of the firm, leading to specialization and the creation of networks and ecosystems (29m13s).
- The limits of a firm's growth are based on a manager's ability, and AI tools could potentially increase the scale of a firm by extending a manager's capabilities (29m38s).
- Parker Conrad, CEO of Rippling, emphasizes the importance of AI's ability to read and process information, allowing managers to extend their capabilities and potentially increase the scale of their firm (30m5s).
- Rippling is attempting to build a suite of HR tools that could potentially replace multiple billion-dollar SaaS companies with one giant company (30m45s).
- The use of AI SaaS tools could give leaders the ability to parse more information and extend the Dunbar number, which is the limit of meaningful relationships humans can have, currently around 150 people (31m12s).
- AI could potentially allow managers to have more meaningful relationships with a larger number of employees, extending the Dunbar limit (31m25s).
- An example of this is a CEO who used a voice chat to call all 1,500 employees, demonstrating the potential for AI to increase a manager's reach and capabilities (31m41s).
- Large language models can have conversations with thousands of people at once, extending the capability of a few individuals to understand what's going on, and this power can be used to extract meaning from conversations and provide summaries (32m16s).
- A product that uses this technology can call up all employees, extract the meaning from their conversations, and provide a bullet point summary to the CEO, which is a significant improvement over traditional SaaS software (32m36s).
- This technology can go beyond just reading and summarizing, and potentially do actual thinking, which raises questions about who is running the organization (33m28s).
- Parker Conrad, the founder of Rippling, is building a team with over a hundred founders who run entire SaaS verticals inside the company, which is a unique approach to building a team (33m49s).
- Rippling is focused on recruiting founders and teams to build on top of the platform, which is similar to Amazon's approach to shared infrastructure (34m29s).
- Every product released by Rippling has hit multi-millions of dollars in ARR on day one, which is a result of having a strong vertical and being able to leverage that to get higher LTV and hold CAC constant (34m45s).
- This approach is an alternative to going from zero to one totally on your own, and companies like Oracle, Microsoft, and Salesforce have used this approach to achieve success (35m6s).
AI voice calling companies (35m22s)
- AI voice calling companies are a rapidly growing subcategory, with companies like Salient using AI to automate voice calls, such as debt collection, which is a tedious task typically performed by low-wage workers in call centers (35m22s).
- Salient has achieved high accuracy in its AI voice calling and is going live with several major banks, demonstrating the potential for AI to automate tasks that were previously performed by humans (36m10s).
- Other companies, such as Vapy, are providing voice infrastructure, making it easier for new companies to enter the market and scale quickly (36m33s).
- Retail companies are also reaching scale quickly, with some companies able to get up and running within hours, which is a testament to the rapid progress being made in the field of AI voice calling (36m40s).
- However, there are still unanswered questions, such as how to hold onto customers, especially with the emergence of new open AI voice APIs, and whether companies can continue to raise the ceiling to keep customers engaged (36m56s).
- The quality of AI voices has improved significantly in the past six months, with voices now sounding more realistic and having lower latency, making them more suitable for replacing human callers (37m35s).
- The development of AI-powered apps has progressed rapidly, from simple text-based apps to more complex vertical AI agents that can replace entire teams and functions within enterprises (39m13s).
- The rate of progress in the field of AI is unprecedented, with significant advancements being made every three months, and the foundation models are becoming increasingly competitive, with companies like Claude emerging as major contenders (39m26s).
- The increasing competition in the market is expected to lead to a more fertile ecosystem, with consumers having more choices and founders having a better chance of success (39m57s).
What is the right vertical for you as a founder? (40m4s)
- To find the right vertical for a startup, it is essential to identify boring, repetitive admin work that can be automated with AI agents, as this common thread is seen across successful companies in this space (40m16s).
- Having direct experience or a relationship with the chosen vertical can be beneficial, as seen in companies that have shown promise (40m37s).
- The company Sweet Spot is building an AI agent to bid on government contracts, an idea they discovered through a friend who had a full-time job refreshing a government website for new proposals to bid on (40m52s).
- Another company, from a recent batch, is building an AI agent to process medical billing for dental clinics, an idea that came from one of the founders working with their mother, a dentist, and identifying the repetitive tasks involved in processing claims (41m13s).
- Identifying the right vertical often involves exploring personal connections and experiences, such as working with family members or friends in a particular industry, to discover areas where AI agents can add value (41m22s).
Outro (41m36s)
- The classic maxim in robotics is that profitable and functioning robots will take on dirty and dangerous jobs, but for vertical SaaS, the focus is on automating boring, repetitive tasks. (41m36s)
- Vertical SaaS aims to automate jobs that are dull and uninteresting, often referred to as "boring butter passing jobs." (41m47s)
- The discussion on vertical AI agents and their potential to be 10 times bigger than SaaS has come to an end. (41m53s)