Why Vertical LLM Agents Are The New $1 Billion SaaS Opportunities
04 Oct 2024 (9 months ago)
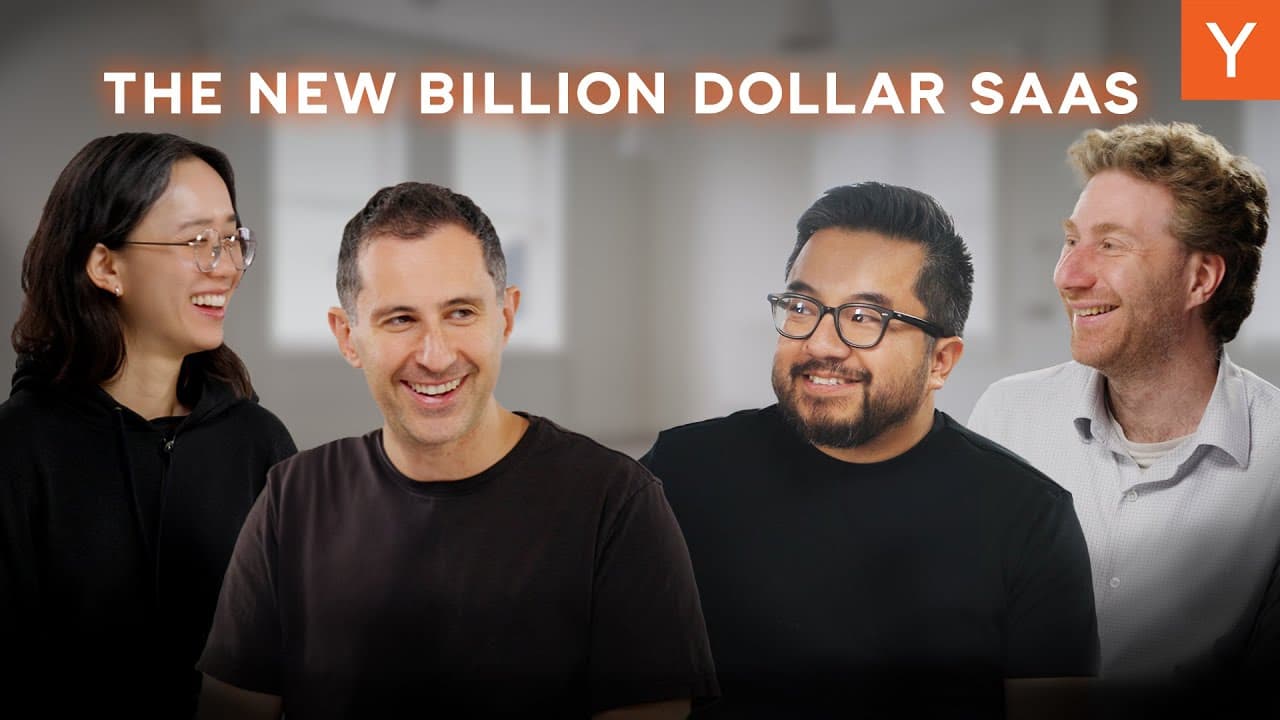
Intro (0s)
- The experience of interacting with a powerful AI for the first time is described as a "Godlike feeling" where tasks that would normally take a whole day are completed in a minute and a half (2s).
- A company of 120 people worked tirelessly for months before the release of GPT-4, feeling like they had an opportunity to get ahead of the market (13s).
- The hosts, Gary and Jared, introduce themselves and mention that Diana Harge is absent but will return for the next episode (30s).
- The guest, Jake Heler of CaseText, is introduced as one of the first people to create value from large language models, with his company going from a $0 to $100 million valuation in 10 years (42s).
- After the release of GPT-4, CaseText's valuation increased to a liquid exit of $650 million in a matter of 2 months, resulting in an acquisition by Thompson Reuters (1m1s).
- Jake Heler is described as one of the first people to realize the potential of large language models and bet his company on it, with successful results (1m23s).
- Jake's story is highlighted as an example of creating real value from large language models, and he is welcomed as a guest to share his lessons and experiences (1m33s).
Building a successful vertical AI company (1m40s)
- Many successful founders are now starting vertical AI agent companies, with dozens of YC companies in the last batch focusing on building vertical-specific AI agents (1m44s).
- Jake, the founder of a successful vertical AI agent company, built his company by leveraging his experience as a lawyer and his computer science training to address the inefficiencies of technology in the legal space (3m52s).
- Jake's company, originally called Casex, was initially focused on annotated versions of case law, but later shifted to building a new product called Co-Counsel based on the GPT-4 technology (3m45s).
- Jake's company had the opportunity to test early versions of GPT-4, and within 48 hours, they decided to shift the entire company's focus to building Co-Counsel, which was a significant change for the 20-person team at the time (3m11s).
- As a lawyer, Jake was frustrated with the technology available for legal research, which involved reading stacks of documents and searching for relevant information in a time-consuming and inefficient process (4m43s).
- Jake's computer science background drove him to build browser plugins and other tools to make his work more efficient, but he eventually left his law firm to start his own company and apply to YC (5m37s).
- Jake's experience as a lawyer and his computer science training gave him a unique perspective on how to apply technology to the legal space, which ultimately led to the development of his successful vertical AI agent company (5m30s).
The unique challenges of law and AI (6m5s)
- The first 10 years of case text were a long slog in the pre-LLM era, and one of the lessons learned was that starting a company may not immediately yield the exact right solution, but rather a general direction that takes time to figure out (6m6s).
- The initial approach to solving the combined issue of bad technology and the need for content in the legal sphere was to create a user-generated content (UGC) site where lawyers could annotate case law and provide information, but this approach failed due to lawyers' valuable time and billing by the hour (6m28s).
- The target audience of lawyers differs significantly from those who contribute to UGC sites like Wikipedia, as lawyers have limited time and bill by the hour, making it difficult to encourage them to contribute to a UGC site (7m12s).
- The company had to pivot and invest in natural language processing and machine learning, which allowed them to automate some of the benefits of their competitors' content databases and create better user experiences (7m29s).
- The early AI-powered features included recommendation algorithms that analyzed case citations and helped lawyers with their work, but these improvements were relatively incremental and easy to ignore for some clients (8m6s).
- Many clients were resistant to change, as they were making a significant amount of money and did not want to introduce anything that could potentially disrupt their workflow or make their life worse, even if it could make them more efficient (8m58s).
The turning point for lawyers with ChatGPT (9m24s)
- The release of ChatGPT marked a turning point for lawyers, as they realized the technology would substantially change their work, even if they weren't sure exactly how (9m32s).
- Lawyers, including those earning high incomes, began to take notice of the potential impact of ChatGPT on their profession and sought to stay ahead of the technology (9m52s).
- The technology itself and market perceptions of what was necessary changed, leading to a fundamental shift that lawyers could no longer ignore (10m21s).
- The concept of the "idea maze" is used to describe the process of startup founders navigating uncertainty and making adjustments, such as pivoting, to reach product-market fit (10m30s).
- The emergence of large language models (LLMs) like ChatGPT shook up the idea maze, bringing some startups closer to product-market fit than others (11m7s).
- The speaker's company was well-positioned to take advantage of this shift, having worked on AI technology, including GPT 4, and having received interest from lawyers and law firms looking to adapt to the changing landscape (10m11s).
Finding product market fit in legal (11m25s)
- The experience of achieving product-market fit is described as a chaotic and intense period, with rapid growth and high demand, as mentioned in an article by Marc Andreessen titled "The Only Thing That Matters," which lists indicators such as servers going down, inability to hire support and sales people fast enough, and extensive media coverage (11m28s).
- When CoCounsel was launched, the company experienced similar chaos, with servers crashing, difficulty hiring support and sales staff, and a surge in media attention, including features in the ABA Journal, CNN, and MSNBC (12m8s).
- The company's AI Legal Assistant, CoCounsel, was developed over a weekend after seeing the potential of GPT-4, and it was designed to be a virtual member of a law firm, capable of tasks such as reading documents, summarizing content, and conducting legal research (12m56s).
- The initial version of CoCounsel was tested with a handful of customers under a non-disclosure agreement (NDA) with OpenAI, and the feedback was overwhelmingly positive, with law firms reporting significant time savings and improved productivity (13m48s).
- The company's intense focus and rapid iteration during the six months leading up to the public launch of GPT-4 allowed them to stay ahead of the market and capitalize on the opportunity, with the entire team working extremely hard to refine the product (14m39s).
- The company's success ultimately led to a $650 million acquisition, with the conversation starting just two months after the launch of CoCounsel, although the transaction did not close until six months later (12m39s).
Entering deep founder mode (15m4s)
- Transitioning a company to adopt a new technology, such as AI, can be challenging, especially when employees are resistant to change due to past experiences with the founder's decisions (15m4s).
- The founder, Jake, had to convince employees and some board members to invest in the new technology, which was a difficult task, especially since the company was already growing at a rate of 70-80% year-over-year and had an ARR of $15-20 million (16m0s).
- To persuade employees, Jake led by example and built the first version of the new product himself, which helped to demonstrate its potential and convince others to get on board (16m21s).
- Initially, only Jake and his co-founder had access to the new product due to NDA restrictions, but this limited access actually helped to build excitement and anticipation among employees (16m41s).
- The company's executives were first introduced to the new product at an executive offsite meeting, where Jake presented the product and shifted the focus away from sales targets and towards the new technology (17m12s).
- Bringing in customers early to test the product and provide feedback also helped to convince skeptical employees of its potential and changed minds quickly (17m34s).
- Seeing customers react positively to the product in real-time, even if it was just over a Zoom call, was a powerful way to demonstrate its value and build excitement among employees (17m44s).
- The reaction of senior attorneys to the capabilities of a new AI model was one of surprise and concern, with some expressing a desire to retire early rather than deal with the implications of the technology (18m17s).
- The development of the AI model was driven in part by the release of GPT-4, which provided access to more advanced language processing capabilities than its predecessors, GPT-2 and GPT-3 (18m24s).
- Initially, the AI model was not suitable for use in legal applications, as it was prone to "hallucinating" or making things up, which is not acceptable in a field where accuracy and facts are crucial (18m54s).
- However, with the release of GPT-3.5, the model showed some promise, with a study indicating that it scored in the 10th percentile on the bar passage, which is better than some human test-takers (19m24s).
- Further testing with GPT-4 showed significant improvement, with the model scoring better than 90% of human test-takers on the bar passage and demonstrating the ability to accurately respond to questions and cite relevant information (19m45s).
- The improvement in the AI model's capabilities was a major turning point, and it marked a shift in the mindset of the researchers and developers working on the project (20m12s).
- The development of the AI model was a collaborative effort between the company and OpenAI, with the two parties working together to test and refine the model's capabilities (19m38s).
Approaching prompt engineering step by step (20m40s)
- The process of approaching prompt engineering involves breaking down a complex task into smaller steps and understanding what the end result should be, with the goal of solving a specific problem for the user (21m14s).
- In the case of legal research, the process involves taking an English language query and breaking it down into search queries, executing the search queries against databases of law, and then compiling the results into a research memo (22m1s).
- The best attorney in the world would approach this problem by breaking down the request into actual search queries, using special search syntax, and then executing the search queries against databases of law (22m3s).
- To accomplish this task using previous technology was impossible, but now it's possible to break down the task into individual prompts, with each prompt thinking step by step (23m15s).
- The process involves writing a series of tests for each prompt, with a clear sense of what good looks like, and using a battery of tests to ensure the prompt is working correctly (23m37s).
- The prompt engineers write English language prompts to try to get the right answer, using a test-driven development approach, which is even more important in the world of prompting due to the unpredictable nature of large language models (LLMs) (24m15s).
- The process involves writing a gold standard answer for each prompt, with a clear sense of what the output should look like, and using this to test the prompt and ensure it's working correctly (24m5s).
- The goal is to get the prompt to work correctly a high percentage of the time, such as 1,200 times out of 1,200, and to use this process to continually improve the prompt and ensure it's working as intended (24m20s).
- The approach to prompt engineering involves thinking step by step, breaking down complex tasks into smaller steps, and using a test-driven development approach to ensure the prompts are working correctly (23m19s).
Going beyond GPT wrappers (25m5s)
- Many companies are not just building GPT wrappers, but are actually adding multiple layers of complexity to solve problems for customers, resulting in full applications that go beyond simple GPT wrappers (25m12s).
- These applications may include proprietary data sets, connections to customer databases, and specific integrations with industry-specific systems, such as legal document management systems (25m40s).
- Even seemingly subtle aspects, such as OCR programs and settings, can be crucial in building a successful application that works well (26m1s).
- Dealing with edge cases and building a robust application that can handle various inputs and scenarios can require dozens of custom-built components (26m27s).
- The prompting piece, including writing tests and specific prompts, and the strategy for breaking down complex problems, also becomes a key part of the application's IP (26m41s).
- This IP is hard to replicate and build, making it a valuable asset for businesses (27m1s).
- Successful SaaS companies often require very specific, custom, and esoteric niche integrations, such as plug-ins to specialized databases (27m12s).
- Many SaaS companies, like Salesforce, built their business logic around databases and connections between tables, and made these accessible to non-technical users (27m26s).
- While demos in chat GPT can be impressive, building an application that works 100% of the time is a much more challenging task that requires significant development and testing (27m46s).
- Customers are often willing to pay a premium for applications that work reliably and efficiently, rather than settling for a 70% solution (27m57s).
Aiming for 100% accuracy (28m10s)
- To achieve 100% accuracy in a mission-critical use case, such as providing information to lawyers working on important court cases, a test-driven development framework was employed, allowing patterns and mistakes to be identified and addressed through the addition of instructions (28m36s).
- The framework involved analyzing why the agent made mistakes, refining instructions, and ensuring the agent had the right amount of information to understand the context, ultimately leading to passing tests and achieving accuracy (29m0s).
- It was found that if the agent passed 100 tests, the likelihood of it performing 100% accurately on the next 100,000 user inputs was very high (29m18s).
- Many founders are tempted to use a "raw dog" approach, relying on prompt engineering without testing, but this method is not suitable for mission-critical applications where accuracy is paramount (29m31s).
- The use case and the need for a "right answer" drove the decision to prioritize accuracy, as lawyers would not tolerate mistakes, and the founder's experience as a lawyer and working with lawyers reinforced this requirement (29m58s).
- The importance of achieving 100% accuracy is not limited to the legal domain, as many fields require high accuracy to maintain trust and faith in the technology (30m21s).
- A single bad experience with an AI system can lead to a loss of faith, making it crucial to ensure the first encounter is successful, especially for non-technologists like busy lawyers (30m29s).
Thoughts on o1’s capabilities (30m48s)
- The current generation of LLMs, such as GBD4, are great at "system one thinking," which is fast and intuitive decision-making based on patterns, but they struggle with executive function, which involves slower and more deliberate thinking (30m51s).
- The newly announced model, AAN, is exciting because it may be able to unlock "system two thinking," which is the missing piece to achieving Artificial General Intelligence (AGI) (31m34s).
- The model "one" is impressive, showing a high degree of thoroughness, precision, and intelligence in its responses, even when given complex tests, such as identifying errors in a 40-page legal brief (31m59s).
- One notable test involved altering a lawyer's quotations in a legal brief to make them incorrect, and then asking the model to identify the errors, which it was able to do successfully, unlike previous LLMs (32m17s).
- The model's ability to think through problems step-by-step, rather than just relying on context, may be due to its training data, which could include a giant corpus of internal monologues of people thinking through problems (33m26s).
- The model's performance may be improved by breaking down complex problems into smaller, more manageable chunks, allowing it to achieve 100% accuracy, rather than relying on a single context window (33m51s).
- It is possible that the model's developers have changed their approach to training data, focusing on how to think about solving problems, rather than just providing input and output (34m5s).
- The current state of large language models (LLMs) is limited by the intelligence of the people writing instructions for them, and researchers are investigating ways to prompt LLMs to think more critically and strategically during their thinking process (34m19s).
- One potential approach is to inject domain expertise or intelligence into the LLM's thinking process, teaching it not just how to answer questions but how to think and approach problems (34m50s).
- This technology has the potential to disrupt various industries, including law, by automating tasks that currently require millions of dollars in salaries and resources (36m0s).
- Companies that develop AI systems capable of performing even a fraction of these tasks can create significant value and unlock new opportunities (36m22s).
- Despite the potential of LLMs, many people still hold misconceptions about their capabilities and limitations, and it's essential to encourage innovation and experimentation in this field (36m31s).
- The development of LLMs is still in its early stages, and there is a need for more research and experimentation to fully realize their potential (34m43s).
- The ability to evaluate and fine-tune LLMs is crucial, and getting to 100% accuracy can provide significant advantages and create new opportunities (35m43s).
- The potential for LLMs to create new billion-dollar companies is significant, and researchers and entrepreneurs should be encouraged to explore this field (35m51s).
- The impact of LLMs can be seen in various industries, and their development can lead to significant improvements in efficiency, productivity, and innovation (36m16s).
Outro (36m42s)
- The jobs that exist today will not disappear, but instead, they will become more interesting in the future (36m43s).
- The conversation has come to an end due to time constraints, and appreciation is expressed to Jake for participating (36m48s).
- The host thanks Jake again and bids farewell to the audience, concluding the session (36m50s).