Stanford CS229 I Machine Learning I Building Large Language Models (LLMs)
29 Aug 2024 (10 months ago)
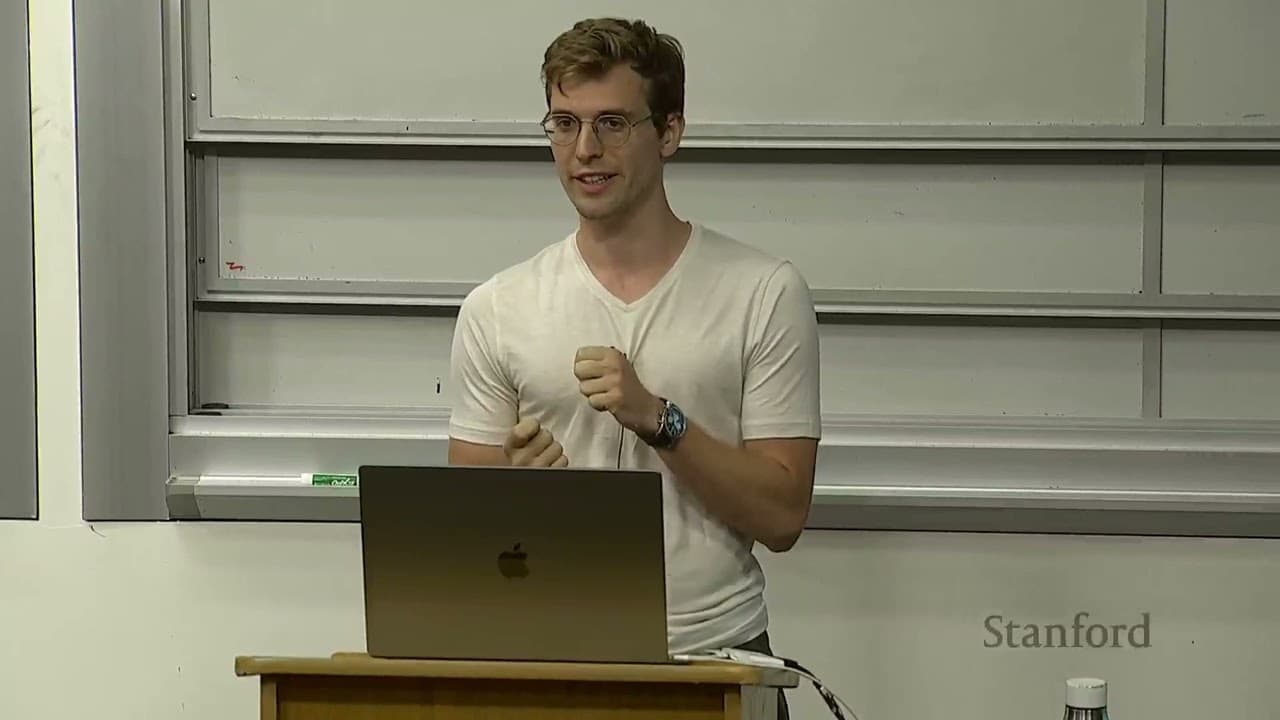
Large Language Models: An Overview
- Large language models (LLMs) like ChatGPT, Claude, and Gemini are essentially chatbots. (16s)
- LLMs are generative models because they can generate sentences by sampling from a probability distribution of word sequences. (4m55s)
- Autoregressive language models decompose the probability distribution of a word sequence into a product of conditional probabilities of each word given the preceding words. (5m19s)
- Autoregressive language models predict the next word or token in a sequence based on the preceding context. (6m0s)
- These models tokenize text, embed tokens into vector representations, process them through a neural network (like a Transformer), and output a probability distribution over potential next tokens. (8m45s)
Tokenization in LLMs
- Tokenization is crucial because it handles variations in language, such as typos, by breaking down words into smaller units, ensuring that even misspelled words can be processed by the model. (10m50s)
- Tokens are more general than words and are not equivalent to words. (11m41s)
- Byte Pair Encoding is a common tokenization algorithm that works by merging the most common pairs of tokens in a large corpus of text. (12m32s)
- When merging tokens during tokenization, the smaller tokens are kept, and each token is assigned a unique ID. (15m21s)
- Tokenization methods: Currently, tokenization is favored over character-by-character or byte-by-byte methods due to length limitations in existing architectures. However, there is hope that future architectures will not have these limitations, leading to a move away from tokenizers. (17m40s)
- Drawbacks of tokenization: One major drawback is the handling of numbers in math. Tokenizing numbers as single units prevents models from understanding and generalizing mathematical concepts effectively. (17m56s)
Evaluating Large Language Models
- Evaluating large language models (LLMs): Perplexity, a measure of how well a model predicts a sequence of words, was commonly used for evaluating LLMs. However, it is no longer favored in academic settings due to its dependence on tokenizers and data. (21m6s)
- MLU, a common benchmark for LLMs, uses a question-and-answer format across various domains like medicine, physics, and astronomy. (22m34s)
- Evaluating open-ended questions in LLMs presents a significant challenge. (25m1s)
- The accuracy of LLM evaluations can be influenced by inconsistencies in evaluation methods and potential contamination of test sets with training data. (26m4s)
- Chatbot Arena is a popular and reliable benchmark for evaluating chatbots, where users interact with and rate different models. (1h30m8s)
- AlpacaEval, an alternative evaluation method, uses LLMs to rate responses from different models and demonstrates a 98% correlation with human evaluations on Chatbot Arena. (1h31m49s)
Training Data for LLMs
- Common Crawl is a web crawler that downloads and stores new websites found by Google every month. This data set contains approximately 250 billion pages and 1 exabyte of data. (29m39s)
- Before using data to train large language models, undesirable content such as NSFW material, harmful content, and personally identifiable information (PII) is filtered out. (31m12s)
- After undesirable content is removed, data is further refined by removing duplication in headers, footers, URLs, and frequently duplicated paragraphs from sources like books. (31m50s)
- High quality websites, such as those linked through Wikipedia, are used to train a classifier to identify high-quality documents. (33m53s)
- Data is classified into domains (entertainment, books, code, etc.) and then weighted to improve model performance in specific areas, such as reasoning. (34m32s)
- The Pile is an academic benchmark for language models that includes data from sources like Wikipedia, GitHub, and books. (39m35s)
Scaling Laws and Training Costs
- Current best language models are trained on approximately 15 trillion tokens, equivalent to 80 exabytes of data. (39m10s)
- The largest language models, such as Lama 2 and Lama 3, are trained on trillions of tokens. (40m10s)
- Scaling laws demonstrate that increasing the amount of data and the size of language models leads to better performance, with predictable improvements in performance based on increases in compute, data, and parameters. (40m55s)
- There are two machine learning pipelines: an older pipeline where hyperparameters are tuned on large models for a short time, and a newer pipeline where a scaling recipe is found by training smaller models of different sizes and extrapolating the results to larger models. (45m8s)
- Scaling laws can be used to determine the optimal allocation of training resources, such as whether to train a larger model on less data or a smaller model on more data. (49m27s)
- The Chinchilla paper demonstrated the use of scaling laws to determine the optimal allocation of training resources by varying the number of tokens and model size while keeping the amount of compute constant. (49m47s)
- The Chinchilla paper suggests an optimal ratio of 20 tokens per parameter for training language models to achieve the lowest loss given a fixed compute budget. (51m37s)
- Considering inference costs, a ratio of approximately 150 tokens per parameter is more practical, as smaller models require less computational resources during inference. (52m35s)
Computational Costs and Environmental Impact
- Estimating the computational cost of training large language models, such as Llama 3 400b, involves considering factors like the number of parameters (45 billion in this case) and the total number of tokens used in training (15.6 tokens for Llama 3 400b). (55m24s)
- The creators of the model aimed to stay below the US government's threshold for special scrutiny, which applies to models with 1e26 flops or more. (56m13s)
- Training the model required approximately 26 million GPU hours over 70 days and cost an estimated $75 million, including compute costs, salaries, and other expenses. (57m2s)
- While significant, the carbon emissions from training the model are considered relatively small compared to future models, estimated to be around 4,000 tons of CO2 equivalent. (58m40s)
Fine-tuning LLMs
- Supervised fine-tuning (SFT) involves fine-tuning a large language model on desired answers collected from humans, essentially performing language modeling on real answers. (1h2m22s)
- SFT does not require a large amount of data, as demonstrated by the Lima paper, which showed that scaling data from 2,000 to 32,000 examples did not significantly improve results. (1h5m31s)
- While synthetic data generation using LLMs is a promising area of research, it is not as crucial for SFT, and obtaining a smaller set of high-quality human-generated data (around 2,000 examples) might be sufficient. (1h7m20s)
- Supervised fine-tuning (SFT) involves training a language model (LLM) on a smaller, specialized dataset using the same language modeling loss function and potentially different hyperparameters. (1h8m51s)
- While humans may be better at distinguishing between good and bad outputs, they may not be the best at generating ideal responses, limiting the effectiveness of behavioral cloning in SFT. (1h10m38s)
Reinforcement Learning from Human Feedback (RLHF)
- Reinforcement learning from human feedback (RLHF) addresses limitations of SFT by having a fine-tuned LLM generate multiple responses for a given instruction, which are then ranked by humans to train a reward model that captures human preferences. (1h12m27s)
- There are two options for reward signals in reinforcement learning for language models: using a binary reward based on human preference between a baseline output and the model's output (1h13m37s), or training a reward model to classify the degree of preference between two outputs (1h14m11s).
- The reward model uses a softmax function to provide continuous reward values, offering more information than a binary reward (1h16m22s).
- The Proximal Policy Optimization (PPO) algorithm is used with a regularization term to prevent over-optimization based on the reward model (1h17m1s).
- Proximal Policy Optimization (PPO) is theoretically appealing but practically messy due to complexities like rollouts, out-of-loop optimization, and clipping. (1h19m7s)
- DPO, a simplification of PPO, proposes maximizing the probability of generating desired outputs and minimizing the probability of generating undesired outputs, essentially maximizing the "green" and minimizing the "red" in human preferences. (1h20m4s)
- While PPO involves training a reward model and using reinforcement learning, DPO simplifies the process to maximum likelihood, making it more straightforward. (1h21m17s)
- Reinforcement learning with human feedback (RLHF) uses a smaller number of tokens, around 1 million, compared to the 15 trillion tokens used in pre-training. (1h34m52s)
Challenges in RLHF
- Humans tend to favor longer responses over shorter ones, even if the shorter response is a better answer. (1h24m27s)
- Labeling data is a complex task, with humans only agreeing with themselves around 66% of the time on a binary task. (1h26m30s)
- Large Language Models (LLMs) are now being used to collect data for Reinforcement Learning from Human Feedback (RLHF), as they are cheaper and have higher agreement with the mode of human responses. (1h27m30s)
- Perplexity, a common metric for evaluating language models, becomes problematic when applied to large language models (LLMs) because these models are not trained to maximize likelihood. (1h29m50s)
Pre-training and Fine-tuning
- Pre-training is viewed as the initialization of a model's weights, and fine-tuning solely on post-training data is what truly trains the model. (1h35m50s)
Hardware and Optimization Techniques
- GPUs (graphics processing units) are optimized for high throughput and fast matrix multiplications, making them suitable for tasks like machine learning. (1h37m55s)
- Using 16 bits instead of 32 bits for floats during matrix multiplication significantly improves processing speed in deep learning contexts. (1h40m45s)
- Automatic mixed precision is a technique where weights are stored in 32 bits for accuracy, but computations are performed in 16 bits for speed. (1h40m50s)
- Operator fusion, achieved through
torch.compile
, optimizes PyTorch code by minimizing data transfers between a GPU's memory and processors, leading to faster computation. (1h42m44s)